Policy Recommendations
Policy Recommendations
As artificial intelligence becomes increasingly embedded in financial decision-making—governing everything from credit approvals and fraud detection to algorithmic trading and systemic risk modeling—current regulatory frameworks remain ill-equipped to ensure transparency, accountability, and security in AI use. The lack of a single, legally defined standard for "explainability" has allowed opaque "black-box" models to make high-stakes decisions without sufficient oversight or justification, undermining consumer trust, raising the risk of discrimination, and eroding legal compliance. Existing guidance such as the NIST AI Risk Management Framework lacks the sector-specific tools necessary to address unique financial threats such as AI-enabled market manipulation and adversarial attacks. Without explainable AI requirements and a finance-specific risk framework, the financial sector faces growing exposure to algorithmic errors, regulatory blind spots, and systemic vulnerabilities—making this an urgent issue for policymakers seeking to protect market integrity, consumer rights, and financial stability in an AI-driven era.
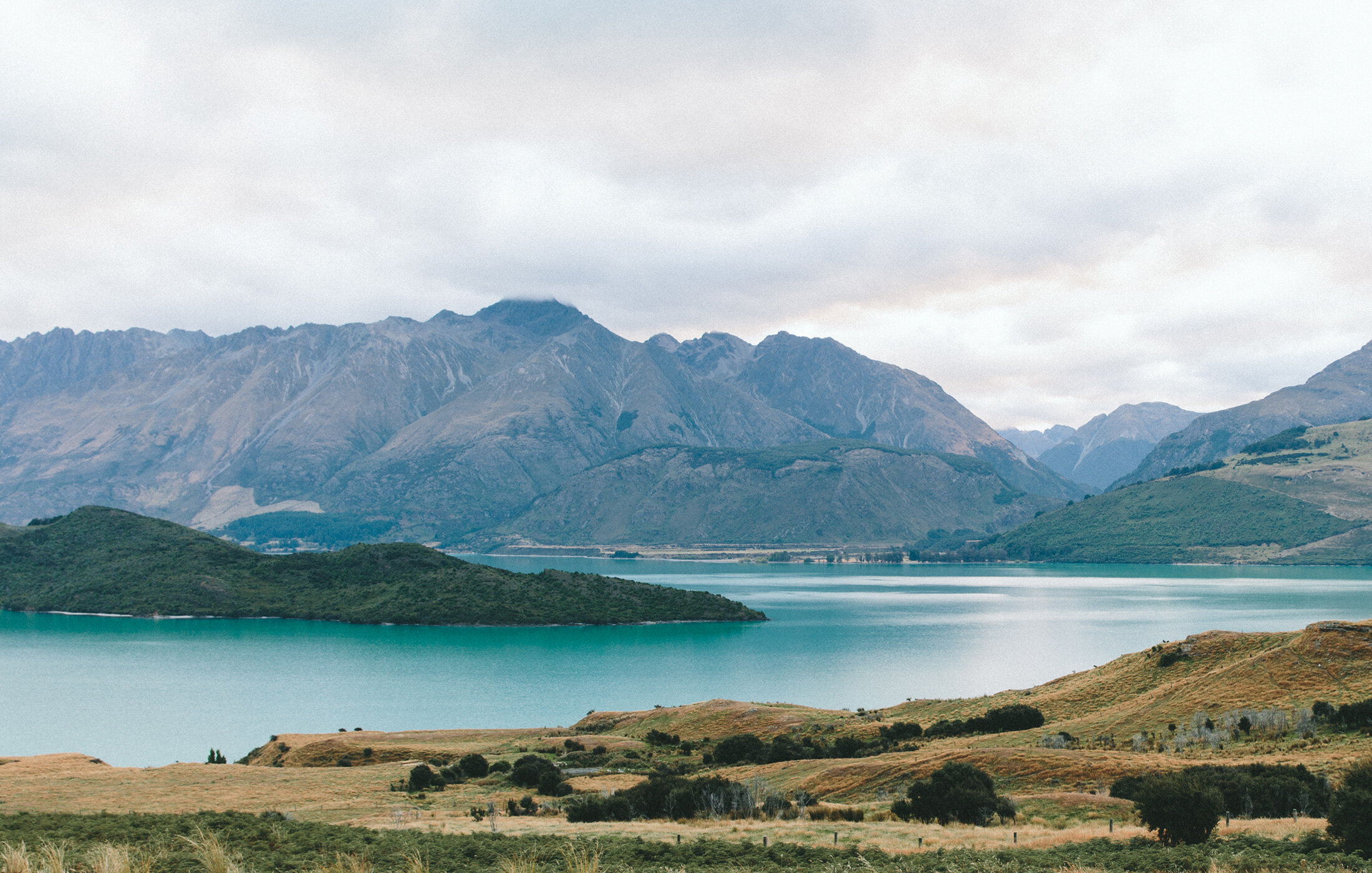
Some industry leaders and policy-makers argue that financial services, due to its existing regulations, is better positioned than other industries when it comes to AI technology. While finance is a heavily regulated industry as is, there are still things stakeholders can do to ensure a future of safe and trustworthy AI use.
-
Explainable AI Standards
Requiring explainability standards means that any AI system used by financial institutions, whether for credit decisions, trading strategies, fraud detection, etc., must produce outcomes that can be understood and explained in human terms. This entails designing or documenting AI models so that their decision-making process can be interpreted by experts and laymen alike. The goal is to avoid "black-box" algorithms whose results cannot be justified or audited . This transparency is crucial in finance to ensure accountability, fairness, and compliance. If an AI model denies a loan or flags a transaction as fraudulent, the institution should be able to articulate which risk factors or rules led to that outcome, rather than hiding behind an opaque algorithm. Lack of explainability undermines customer trust, poses legal risks (such as violating anti-discrimination laws), and safety risks (undetected errors or biases). This policy aims to make AI decisions traceable and reviewable, aligning with existing financial regulations that require transparency in decision-making.
-
Treasury x NIST AI Risk Management Framework
A collaborative financial-sector-specific AI Risk Management Framework developed by NIST and the U.S. Treasury is essential given the high stakes and vast use cases of applying AI in finance. Financial models directly influence credit access, investment decisions, fraud prevention, and systemic risk exposure, where errors or adversarial attacks can cascade rapidly across institutions and markets. The NIST AI Risk Management Framework (released in 2023) provides a solid foundation, but its broad focus lacks sector-specific guidance on threats like market manipulation via AI trading bots or adversarial attacks on credit and underwriting models. A tailored framework would bridge this gap by offering threat models, red-teaming protocols, and real-world use cases relevant to banks, insurers, and asset managers, helping institutions integrate cybersecurity and fairness controls into every layer of AI deployment.
Moreover, the U.S. Treasury, through agencies like the Office of Financial Research (OFR) and Financial Crimes Enforcement Network (FinCEN), already monitors technological vulnerabilities in the financial system and is well-positioned to assess macroprudential AI risks. A joint NIST-Treasury report could formalize sector-specific best practices for secure model development, documentation, adversarial testing, and third-party vendor oversight, creating a shared baseline for compliance and resilience. It would also encourage cross-agency alignment, helping ensure that regulatory efforts by the Federal Reserve, OCC, and SEC are grounded in a consistent, technically sound framework. As AI use deepens across the financial ecosystem, such a collaboration would not only mitigate cybersecurity risks but also promote responsible innovation by giving firms clear, actionable guidance tailored to the financial industry’s unique risk landscape.
Explainable AI Standards
The challenges lie in implementation details – ensuring that explanations are meaningful, training examiners in AI technical review, and coordinating across many agencies and sectors. However, the legal and regulatory tools to do this are largely in place, from consumer protection laws like ECOA to the supervisory powers of bank and market regulators.
This policy can be implemented through a combination of formal rule-making, supervisory guidance, legal enforcement, model governance, audits, and public disclosure. Three possible enforcement mechanisms are outlined below.
Mechanism One: Model Documentation + Explainability Reports
Banks already follow the SR 11-7 guidance on model risk management. Explainability could be added as a required feature, especially for high-impact models.
Agencies might require documentation of how model decisions can be interpreted and challenged.
This documentation could detail:
How the model works: What input features it uses, its underlying assumptions, and its decision-making logic.
Data Sources and Quality: Details about the datasets used to train and validate the model.
Performance Metrics: Accuracy, bias, error rates, and other performance indicators.
Risk Assessments: Potential risks associated with misclassification or opaque decisions.
Mechanism Three: public-private partnership certification program
Agencies could collaborate with NIST or private standard-setting bodies to develop certification criteria for AI explainability and trustworthiness.
Firms would then be required to use audited and certified AI systems in critical operations.
Relevant Regulators
Several US regulatory agencies (OCC, Federal Reserve, SEC, CFPB, FDIC, NCUA, CFTC, State Insurance regulators) would enforce AI explainability requirements, each within their jurisdiction.
For example, bank regulators could require any AI system used for risk modeling or loan approvals to be validated by an independent third party.
The CFPB could require that any AI used in consumer lending (e.g., credit scoring, loan approval) provide human-understandable reasons for decisions under the Equal Credit Opportunity Act (ECOA).
The SEC could mandate explainability for algorithmic trading tools under investor protection mandates.
Regulators can issue non-binding guidance that sets expectations, especially for systemically important institutions. For example:
The Federal Reserve might issue guidance to banks suggesting that AI models used for credit risk or capital planning meet specific interpretability benchmarks (like SHAP, LIME, or counterfactual analysis).
Mechanism Two: Third Party Audits
Auditors will review AI models during exams – asking firms to demonstrate how the model works, what variables it uses, and to produce explanation examples for certain outputs. For example, examiners might select a set of loan files and ask a bank to show the reasons the AI gave those applicants lower credit scores or higher interest rates, checking that those reasons are reasonable and legally permissible. If a firm cannot explain its AI’s output, regulators can issue findings requiring corrective action. Likewise, FINRA and SEC examiners could inspect a brokerage’s trading algorithms; if the firm’s staff themselves do not understand how the AI is making decisions, that would be a red flag. Over time, failing to maintain explainable models could lead to enforcement actions (fines, limitations on using the model, etc.), especially if it results in consumer harm or regulatory violations.
Legal Feasibility
Most financial regulators already have authority to to require third party audits as part of
Risk management supervision
Internal control verification
Model validation
Compliance with disclosure or fairness laws
This can be done through:
Formal rulemaking (binding requirements)
Supervisory guidance (non-binding but enforced in practice)
Enforcement actions or consent orders (to correct bad behavior)
Public Comment and Stakeholder Engagement
Agencies can issue proposed rules regarding explainability standards and invite public comment, ensuring that industry, consumer advocates, and technical experts have input. This collaborative process can help refine standards that are both rigorous and feasible for implementation.
Counterarguments
*
Counterarguments *
Compliance Burdens
Developing explainable models or retrofitting existing systems with interpretable layers requires specialized talent and substantial resources. Large banks may absorb these costs, but they can disproportionately impact smaller players, exacerbating market consolidation. According to a paper by the Journal of Next-Generation Research, “For small and medium-sized enterprises (SMEs), these requirements pose considerable challenges due to limited resources and expertise.” Critics also note that "explainability" is not always well-defined or universally applicable across financial use cases. What is understandable in consumer lending might be meaningless or infeasible in high-frequency trading environments. Applying explainability standards across sectors may result in differing interpretations and uncertainty in implementation. These concerns highlight the need for any explainability mandate to be both risk-sensitive and sector-specific, with room for flexibility and proportionality.
Stifle innovation
A strong counterargument to mandatory explainability standards in financial AI is that they could stifle innovation and reduce the effectiveness of high-performing models. Many of the most powerful AI systems, particularly deep learning models, derive their predictive power from complexity, not simplicity. Imposing explainability requirements may force financial institutions to forgo these sophisticated models in favor of more interpretable but less accurate alternatives. This trade-off could impair fraud detection systems, risk modeling, and algorithmic trading strategies that rely on subtle, nonlinear patterns in massive datasets. A report by the Institute of Electrical and Electronics Engineers notes that “high performance models are often less interpretable and most explainable ones have low accuracy,” particularly in complex domains like finance and healthcare. Critics argue that if these standards are too rigid or broadly applied, they risk weakening the very capabilities that make AI such a valuable tool in finance.
While concerns about reduced model performance and compliance burdens are valid, they do not outweigh the fundamental need for explainability in financial AI systems, particularly given the high stakes of decisions involving consumer rights, market stability, and legal accountability. The goal of explainability standards is not to eliminate complexity, but to ensure transparency and trust in critical decisions. Advances in the field of interpretable machine learning have already produced methods that balance accuracy with transparency—such as SHAP values, LIME, and surrogate models—that can be layered on top of complex models to provide meaningful insights without sacrificing performance (Molnar, "Interpretable Machine Learning"). Sector-specific and risk-based approaches, already standard in financial regulation, can ensure flexibility, tailoring explainability requirements to the sensitivity of use cases. For example, a credit denial must be explainable to a consumer, whereas model transparency in high-frequency trading may only need to satisfy expert oversight. Rather than being a barrier to innovation, explainability can enable safer, fairer AI deployment by helping institutions detect bias, prevent errors, and maintain regulatory compliance. In this light, requiring explainable AI is not only a safeguard but a prerequisite for responsible AI adoption in finance.
Explainability: Practical Implementation
Explainability: Practical Implementation
Banking
Banks would incorporate explainability into their model governance. Today, banks follow interagency model risk management guidance that calls for model validation and documentation. Under the new standards, whenever a bank develops or adopts an AI model for a critical function (say, a credit scoring model or a trading algorithm in its treasury operations), the bank’s risk managers must ensure the model can produce understandable output and reason codes. Larger banks, which often develop more advanced AI, might even have AI ethics or oversight committees to ensure models are explainable and fair. Smaller community banks might rely on vendor-provided models, but they would still need to obtain sufficient documentation from the vendor to explain outcomes to customers and regulators.
Asset Management
Asset managers would likely need to beef up their compliance technology teams. If a trading firm uses an AI algorithm to execute trades, it must have a way to audit that algorithm’s decisions after the fact (for instance, was a sudden burst of trades triggered by a specific market signal that the AI learned?). We could see practices like pre-approval of algos by internal risk committees and ongoing monitoring. The SEC and FINRA might ask firms for algorithmic trading risk reports documenting how their AI works and what safeguards ensure it doesn’t violate rules (for example, by inadvertently front-running the market or causing a liquidity crisis). Asset managers using AI for portfolio selection would need to document how those models align with investment objectives. Importantly, if clients ask why a certain investment decision was made by an AI-driven robo-advisor, the firm should be able to give a cogent answer (ex: “the model recommended this fund due to the client’s stated risk tolerance and the fund’s past stability in downturns”). In examinations, the SEC could test a robo-advisor by inquiring about certain recommendations and expecting the firm to produce an explanation for each. The Financial Industry Regulatory Authority (FINRA) would likely update its examination checklist to include AI systems oversight, checking that member firms can explain their AI outputs and have not delegated their regulatory responsibilities entirely to algorithms (American Progress).
Insurance
Insurers would implement explainability through their AI governance programs as prompted by the NAIC Model Bulletin. In practice, an insurance company using an AI model to set prices would keep a technical document explaining the factors (age, location, driving record, etc.) and their influence on rates. If a regulator or consumer inquiry comes in about why a certain policy was priced high, the insurer’s compliance team should be able to trace it to, say, the individual’s accident history carrying a certain weight in the model. Periodically, insurers might have to file a certification or attestation to state regulators that their AI models have been reviewed for explainability and bias. If an insurer uses third-party data or algorithms (for example, an external service that predicts health risks), regulators would expect the insurer to have vetted that service for transparency. Some states might even require insurers to provide consumers an explanation for adverse decisions (similar to credit adverse action notices) – ex: if coverage is denied by an AI, a notice citing the main reasons (which forces the AI to be interpretable). Though insurance regulation varies state by state, the NAIC’s guidance provides a common blueprint emphasizing transparency, fairness, and accountability in AI use.
Treasury x NIST AI Risk Management Framework
Why:
Currently, there is a “lack of clarity as to how financial regulators’ standards and expectations use the NIST AI RMF, if at all, and whether the NIST AI RMF is aligned with prudential or other regulatory expectations related to AI.” (Treasury Dept)
The government needs to create a finance-specifc version of the NIST RMF from 2023 that firms can use to guide their AI governance depending on the existing regulations applicable to their sector.
Feasibility
Government agencies and departments have collaborated with stakeholders in relevant industries to create reports of this nature before, so this is very much in the scope of what precedent dictates.
Notably, CISA is currently working on a set of Sector Specific Goals (SSGs) for the Financial Services Sector. SSGs are developed in partnership with Sector Risk Management Agencies (SRMAs) and sector stakeholders, and they address unique requirements in select critical infrastructure sectors. The financial services version is expected in winter 2025. The AI Risk Profile cybersecurity portion could complement CISA’s SSGs.
How:
The Treasury Department and NIST should utilize conferences, meetings, interviews with stakeholders as well as Requests For Information to ensure all stakeholders’ perspectives are taken into accord. Government agencies and financial services sector should continue finance-specific AI information sharing through forums, publications, research programs, etc.
Who:
Spearheaded collaboration between NIST and the Treasury Department, with the inclusion of diverse stakeholders such as industry representatives, academics, consumer advocates, civil rights groups, technology companies, etc.
Counterarguments
A compelling counterargument to creating a collaborative, financial-sector-specific AI Risk Management Framework is that it may lead to regulatory redundancy and increased compliance burdens without delivering commensurate value. Critics might argue that existing frameworks—such as the NIST AI RMF, the Federal Reserve’s SR 11-7 on model risk, and sector-specific cybersecurity guidance from agencies like the OCC and SEC—already cover much of the necessary ground. Introducing a new framework could overwhelm smaller financial institutions with overlapping standards, diverting resources from implementation to paperwork.
While these concerns are valid, the US Treasury’s report on the Uses, Opportunities, and Risks of Artificial Intelligence surveyed over 100 stakeholders and what emerged was overwhelming support for an alignment of definitions of AI models and systems applicable to the sector, clarity on standards for data privacy, security, and quality for financial firms using AI, and clarity on how to ensure uniform compliance with existing regulations. This response to the Treasury’s Request For Information shows that a report like this providing enumerating best practices and standards is needed.